Collection:
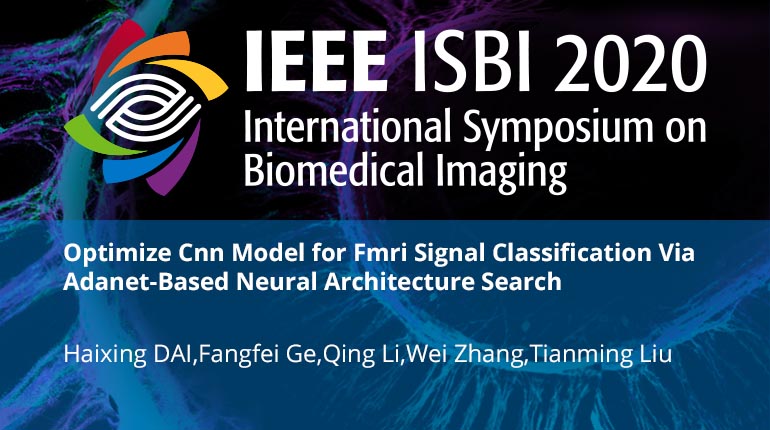
Recent studies showed that convolutional neural network (CNN) models possess remarkable capability of differentiating and characterizing fMRI signals from cortical gyri and sulci. In addition, visualization and analysis of the filters in the learned CNN models suggest that sulcal fMRI signals are more diverse and have higher frequency than gyral signals. However, it is not clear whether the gyral fMRI signals can be further divided into sub-populations, e.g., 3-hinge areas vs 2-hinge areas. It is also unclear whether the CNN models of two classes (gyral vs sulcal) classification can be further optimized for three classes (3-hinge gyral vs 2-hinge gyral vs sulcal) classification. To answer these questions, in this paper, we employed the AdaNet framework to design a neural architecture search (NAS) system for optimizing CNN models for three classes fMRI signal classification. The core idea is that AdaNet adaptively learns both the optimal structure of the CNN network and its weights so that the learnt CNN model can effectively extract discriminative features that maximize the classification accuracies of three classes of 3-hinge gyral, 2-hinge gyral and sulcal fMRI signals. We evaluated our framework on the Autism Brain Imaging Data Exchange (ABIDE) dataset, and experiments showed that our framework can obtained significantly better results, in terms of both classification accuracy and extracted features.
- IEEE MemberUS $11.00
- Society MemberUS $0.00
- IEEE Student MemberUS $11.00
- Non-IEEE MemberUS $15.00
Videos in this product
Optimize Cnn Model for Fmri Signal Classification Via Adanet-Based Neural Architecture Search
Recent studies showed that convolutional neural network (CNN) models possess remarkable capability of differentiating and characterizing fMRI signals from cortical gyri and sulci. In addition, visualization and analysis of the filters in the learned CNN models suggest that sulcal fMRI signals are more diverse and have higher frequency than gyral signals. However, it is not clear whether the gyral fMRI signals can be further divided into sub-populations, e.g., 3-hinge areas vs 2-hinge areas. It is also unclear whether the CNN models of two classes (gyral vs sulcal) classification can be further optimized for three classes (3-hinge gyral vs 2-hinge gyral vs sulcal) classification. To answer these questions, in this paper, we employed the AdaNet framework to design a neural architecture search (NAS) system for optimizing CNN models for three classes fMRI signal classification. The core idea is that AdaNet adaptively learns both the optimal structure of the CNN network and its weights so that the learnt CNN model can effectively extract discriminative features that maximize the classification accuracies of three classes of 3-hinge gyral, 2-hinge gyral and sulcal fMRI signals. We evaluated our framework on the Autism Brain Imaging Data Exchange (ABIDE) dataset, and experiments showed that our framework can obtained significantly better results, in terms of both classification accuracy and extracted features.