Collection:
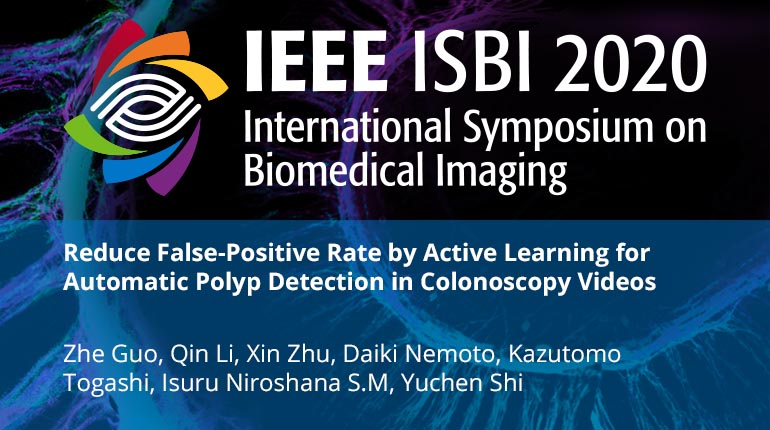
Automatic polyp detection is reported to have a high false-positive rate (FPR) because of various polyp-like structures and artifacts in complex colon environment. An efficient polyp?s computer-aided detection (CADe) system should have a high sensitivity and a low FPR (high specificity). Convolutional neural networks have been implemented in colonoscopy-based automatic polyp detection and achieved high performance in improving polyp detection rate. However, complex colon environments caused excessive false positives are going to prevent the clinical implementation of CADe system. To reduce false positive rate, we proposed an automatic polyp detection algorithm, combined with YOLOv3 architecture and active learning. This algorithm was trained with colonoscopy videos/ images from 283 subjects. Through testing with 100 short and 9 full colonoscopy videos, the proposed algorithm shown FPR of 2.8% and 1.5%, respectively, similar sensitivities of expert endoscopists.
- IEEE MemberUS $11.00
- Society MemberUS $0.00
- IEEE Student MemberUS $11.00
- Non-IEEE MemberUS $15.00
Videos in this product
Reduce False-Positive Rate by Active Learning for Automatic Polyp Detection in Colonoscopy Videos
Automatic polyp detection is reported to have a high false-positive rate (FPR) because of various polyp-like structures and artifacts in complex colon environment. An efficient polyp?s computer-aided detection (CADe) system should have a high sensitivity and a low FPR (high specificity). Convolutional neural networks have been implemented in colonoscopy-based automatic polyp detection and achieved high performance in improving polyp detection rate. However, complex colon environments caused excessive false positives are going to prevent the clinical implementation of CADe system. To reduce false positive rate, we proposed an automatic polyp detection algorithm, combined with YOLOv3 architecture and active learning. This algorithm was trained with colonoscopy videos/ images from 283 subjects. Through testing with 100 short and 9 full colonoscopy videos, the proposed algorithm shown FPR of 2.8% and 1.5%, respectively, similar sensitivities of expert endoscopists.