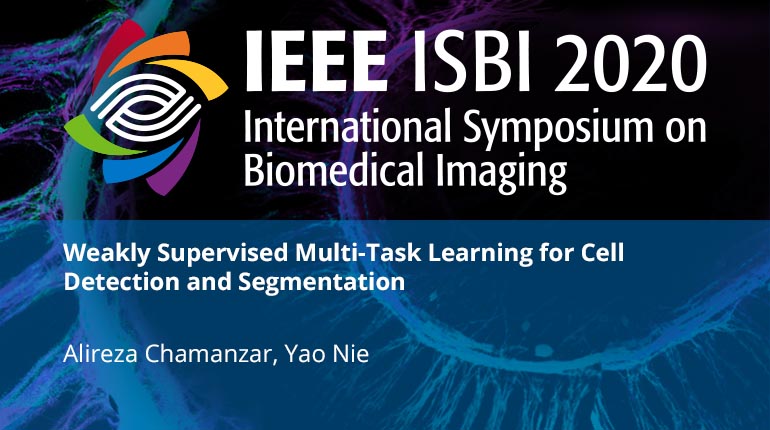
Already purchased this program?
Login to View
This video program is a part of the Premium package:
Weakly Supervised Multi-Task Learning for Cell Detection and Segmentation
- IEEE MemberUS $11.00
- Society MemberUS $0.00
- IEEE Student MemberUS $11.00
- Non-IEEE MemberUS $15.00
Weakly Supervised Multi-Task Learning for Cell Detection and Segmentation
Cell detection and segmentation is fundamental for all downstream analysis of digital pathology images. However, obtaining the pixel-level ground truth for single cell segmentation is extremely labor intensive. To overcome this challenge, we developed an end-to-end deep learning algorithm to perform both single cell detection and segmentation using only point labels. This is achieved through the combination of different task orientated point label encoding methods and a multi-task scheduler for training. We apply and validate our algorithm on PMS2 stained colon rectal cancer and tonsil tissue images. Compared to the state-of-the-art, our algorithm shows significant improvement in cell detection and segmentation without increasing the annotation efforts.
Cell detection and segmentation is fundamental for all downstream analysis of digital pathology images. However, obtaining the pixel-level ground truth for single cell segmentation is extremely labor intensive. To overcome this challenge, we developed an end-to-end deep learning algorithm to perform both single cell detection and segmentation using only point labels. This is achieved through the combination of different task orientated point label encoding methods and a multi-task scheduler for training. We apply and validate our algorithm on PMS2 stained colon rectal cancer and tonsil tissue images. Compared to the state-of-the-art, our algorithm shows significant improvement in cell detection and segmentation without increasing the annotation efforts.