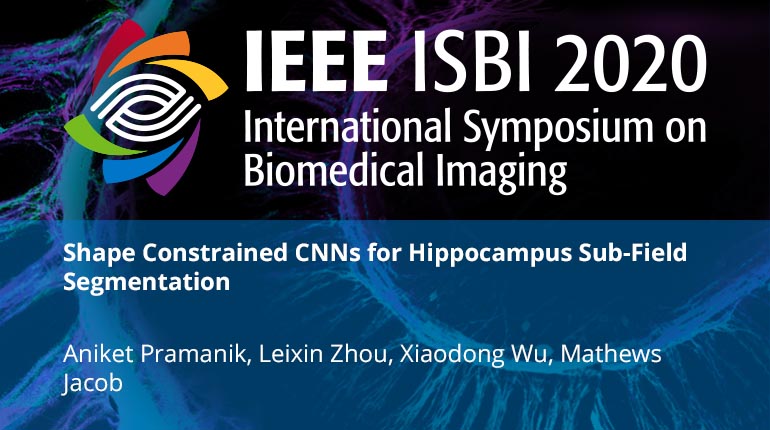
Already purchased this program?
Login to View
This video program is a part of the Premium package:
Shape Constrained CNNs for Hippocampus Sub-Field Segmentation
- IEEE MemberUS $11.00
- Society MemberUS $0.00
- IEEE Student MemberUS $11.00
- Non-IEEE MemberUS $15.00
Shape Constrained CNNs for Hippocampus Sub-Field Segmentation
The segmentation of hippocampus subfields from MR images is challenging due to the small size of the organ, multiple interconnected regions, and low contrast. Motivated by the success of active shape models in segmentation, we propose a shape constrained CNN algorithm. We use a two-step strategy, where we first use an auto-encoder CNN to learn the anatomical shape priors from labels. After shape training, the decoder compactly represents the shape manifold; it generates the labels from a compact code. We then train an encoder CNN to predict the code from MR images. The decoder (shape generator) is fixed during the segmentation training.
The segmentation of hippocampus subfields from MR images is challenging due to the small size of the organ, multiple interconnected regions, and low contrast. Motivated by the success of active shape models in segmentation, we propose a shape constrained CNN algorithm. We use a two-step strategy, where we first use an auto-encoder CNN to learn the anatomical shape priors from labels. After shape training, the decoder compactly represents the shape manifold; it generates the labels from a compact code. We then train an encoder CNN to predict the code from MR images. The decoder (shape generator) is fixed during the segmentation training.