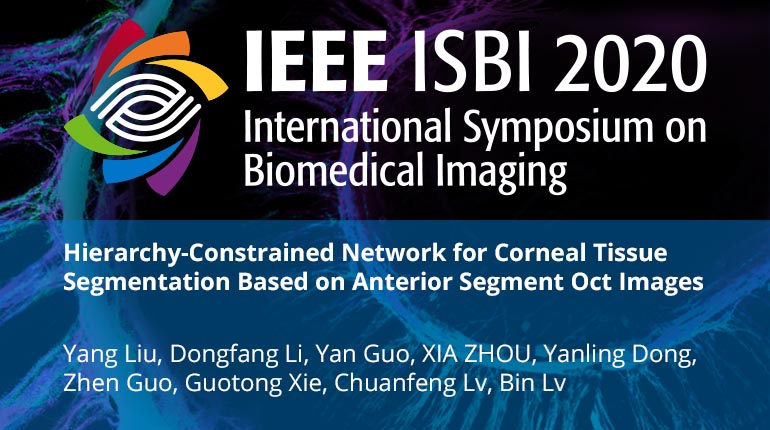
Already purchased this program?
Login to View
This video program is a part of the Premium package:
Hierarchy-Constrained Network for Corneal Tissue Segmentation Based on Anterior Segment Oct Images
- IEEE MemberUS $11.00
- Society MemberUS $0.00
- IEEE Student MemberUS $11.00
- Non-IEEE MemberUS $15.00
Hierarchy-Constrained Network for Corneal Tissue Segmentation Based on Anterior Segment Oct Images
Anterior segment optical coherence tomography (AS-OCT) is widely used to observe the corneal tissue structures in clinical ophthalmology. Accurate segmentation of corneal tissue interfaces is essential for corneal diseases diagnosis and surgical planning. However, image scattered noise and keratopathy make corneal tissue interface fitting results of the existing methods deviate. In this paper, we propose a hierarchy-constrained network, which combines hierarchical features by using of an elegant progressive feature-extraction module and boundary constraint to overcome these challenges. In the meantime, multi-level prediction fusion module is integrated to the network that eventually enables the output node to sufficiently absorb features extracted from various levels. Extensive experimental results on two datasets containing cornea images with multiple lesions show that our proposed method distinctly improves the accuracy of corneal tissue interfaces segmentation and outperforms other existing methods.
Anterior segment optical coherence tomography (AS-OCT) is widely used to observe the corneal tissue structures in clinical ophthalmology. Accurate segmentation of corneal tissue interfaces is essential for corneal diseases diagnosis and surgical planning. However, image scattered noise and keratopathy make corneal tissue interface fitting results of the existing methods deviate. In this paper, we propose a hierarchy-constrained network, which combines hierarchical features by using of an elegant progressive feature-extraction module and boundary constraint to overcome these challenges. In the meantime, multi-level prediction fusion module is integrated to the network that eventually enables the output node to sufficiently absorb features extracted from various levels. Extensive experimental results on two datasets containing cornea images with multiple lesions show that our proposed method distinctly improves the accuracy of corneal tissue interfaces segmentation and outperforms other existing methods.