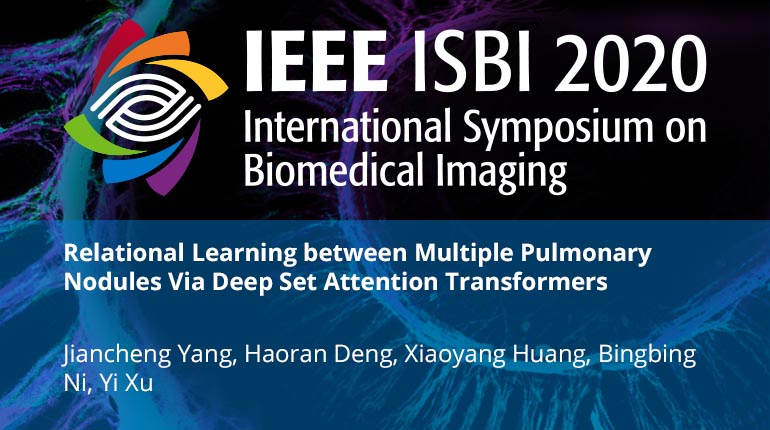
Already purchased this program?
Login to View
This video program is a part of the Premium package:
Relational Learning between Multiple Pulmonary Nodules Via Deep Set Attention Transformers
- IEEE MemberUS $11.00
- Society MemberUS $0.00
- IEEE Student MemberUS $11.00
- Non-IEEE MemberUS $15.00
Relational Learning between Multiple Pulmonary Nodules Via Deep Set Attention Transformers
Diagnosis and treatment of multiple pulmonary nodules are clinically important but challenging. Prior studies on nodule characterization use solitary-nodule approaches on multiple nodular patients, which ignores the relations between nodules. In this study, we propose a multiple instance learning (MIL) approach and empirically prove the benefit to learn the relations between multiple nodules. By treating the multiple nodules from a same patient as a whole, critical relational information between solitary-nodule voxels is extracted. To our knowledge, it is the first study to learn the relations between multiple pulmonary nodules. Inspired by recent advances in natural language processing (NLP) domain, we introduce a self-attention transformer equipped with 3D CNN, named NoduleSAT, to replace typical pooling-based aggregation in multiple instance learning. Extensive experiments on lung nodule false positive reduction on LUNA16 database, and malignancy classification on LIDC-IDRI database, validate the effectiveness of the proposed method.
Diagnosis and treatment of multiple pulmonary nodules are clinically important but challenging. Prior studies on nodule characterization use solitary-nodule approaches on multiple nodular patients, which ignores the relations between nodules. In this study, we propose a multiple instance learning (MIL) approach and empirically prove the benefit to learn the relations between multiple nodules. By treating the multiple nodules from a same patient as a whole, critical relational information between solitary-nodule voxels is extracted. To our knowledge, it is the first study to learn the relations between multiple pulmonary nodules. Inspired by recent advances in natural language processing (NLP) domain, we introduce a self-attention transformer equipped with 3D CNN, named NoduleSAT, to replace typical pooling-based aggregation in multiple instance learning. Extensive experiments on lung nodule false positive reduction on LUNA16 database, and malignancy classification on LIDC-IDRI database, validate the effectiveness of the proposed method.