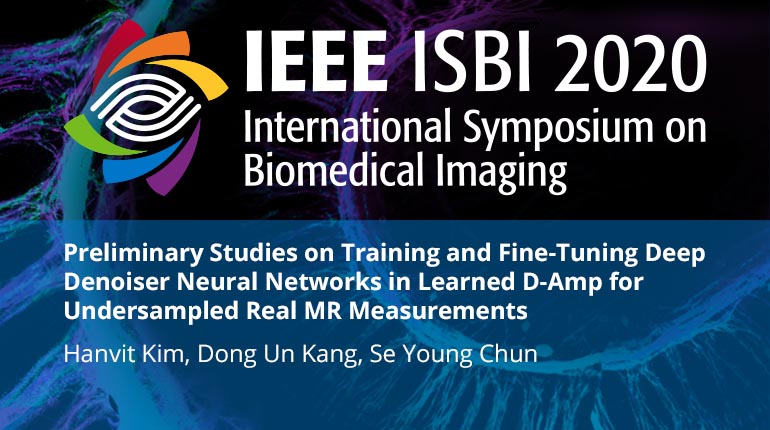
Already purchased this program?
Login to View
This video program is a part of the Premium package:
Preliminary Studies on Training and Fine-Tuning Deep Denoiser Neural Networks in Learned D-Amp for Undersampled Real MR Measurements
- IEEE MemberUS $11.00
- Society MemberUS $0.00
- IEEE Student MemberUS $11.00
- Non-IEEE MemberUS $15.00
Preliminary Studies on Training and Fine-Tuning Deep Denoiser Neural Networks in Learned D-Amp for Undersampled Real MR Measurements
We investigated learned denoiser-based approximate message passing (LDAMP) with undersampled real MR measurements. In our preliminary results, LDAMP yielded favorable performance over BM3D-based AMP even though ground truth images are noisy and deep denoisers were trained only for Gaussian noise, not for undersampling artifacts. We further investigated the feasibility of using Stein?s unbiased risk estimator (SURE) to fine-tune deep denoisers with given undersampled MR measurement to reconstruct. Even though slight performance improvements (0.04dB) were observed for an example case, no visual improvement was observed.
We investigated learned denoiser-based approximate message passing (LDAMP) with undersampled real MR measurements. In our preliminary results, LDAMP yielded favorable performance over BM3D-based AMP even though ground truth images are noisy and deep denoisers were trained only for Gaussian noise, not for undersampling artifacts. We further investigated the feasibility of using Stein?s unbiased risk estimator (SURE) to fine-tune deep denoisers with given undersampled MR measurement to reconstruct. Even though slight performance improvements (0.04dB) were observed for an example case, no visual improvement was observed.