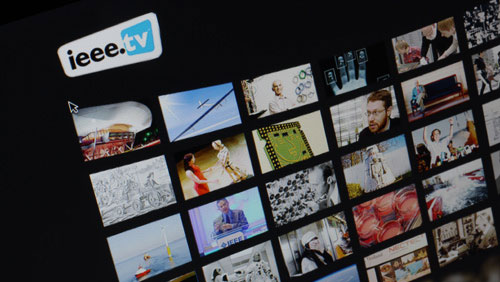
Showing 301 - 350 of 459
- IEEE MemberUS $11.00
- Society MemberUS $0.00
- IEEE Student MemberUS $11.00
- Non-IEEE MemberUS $15.00
Learning to Solve Inverse Problems in Imaging
Many challenging image processing tasks can be described by an ill-posed linear inverse problem: deblurring, deconvolution, tomographic reconstruction, MRI reconstruction, inpainting, compressed sensing, and superresolution all lie in this framework. Traditional inverse problem solvers minimize a cost function consisting of a data-fit term, which measures how well an image matches the observations, and a regularizer, which reflects prior knowledge and promotes images with desirable properties like smoothness. Recent advances in machine learning and image processing have illustrated that it is often possible to learn a regularizer from training data that can outperform more traditional regularizers. Recent advances in machine learning have illustrated that it is often possible to learn a regularizer from training data that can outperform more traditional regularizers. In this talk, I will describe various classes of approaches to learned regularization, ranging from generative models to unrolled optimization perspectives, and explore their relative merits and sample complexities. We will also explore the difficulty of the underlying optimization task and how learned regularizers relate to oracle estimators.
- IEEE MemberUS $11.00
- Society MemberUS $0.00
- IEEE Student MemberUS $11.00
- Non-IEEE MemberUS $15.00
Learning a Loss Function for Segmentation: A Feasibility Study
When training neural networks for segmentation, the Dice loss is typically used. Alternative loss functions could help the networks achieve results with higher user acceptance and lower correction effort, but they cannot be used directly if they are not differentiable. As a solution, we propose to train a regression network to approximate the loss function and combine it with a U-Net to compute the loss during segmentation training. As an example, we introduce the contour Dice coefficient (CDC) that estimates the fraction of contour length that needs correction. Applied to CT bladder segmentation, we show that a weighted combination of Dice and CDC loss improves segmentations compared to using only Dice loss, with regard to both CDC and other metrics.
- IEEE MemberUS $11.00
- Society MemberUS $0.00
- IEEE Student MemberUS $11.00
- Non-IEEE MemberUS $15.00
Automated Left Atrial Segmentation from Magnetic Resonance Image Sequences Using Deep Convolutional Neural Network with Autoencoder
This study presents a novel automated algorithm to segment the left atrium (LA) from 2, 3 and 4-chamber long-axis cardiac cine magnetic resonance image (MRI) sequences using deep convolutional neural network (CNN). The objective of the segmentation process is to delineate the boundary between myocardium and endocardium and exclude the mitral valve so that the results could be used for generating clinical measurements such as strain and strain rate. As such, the segmentation needs to be performed using open contours, a more challenging problem than the pixel-wise semantic segmentation performed by existing machine learning-based approaches such as U-net. The proposed neural net is built based on pre-trained CNN Inception V4 architecture, and it predicts a compressed vector by applying a multi-layer autoencoder, which is then back-projected into the segmentation contour of the LA to perform the delineation using open contours. Quantitative evaluations were performed to compare the performances of the proposed method and the current state-of-the-art U-net method. Both methods were trained using 6195 images acquired from 80 patients and evaluated using 1515 images acquired from 20 patients where the datasets were obtained retrospectively, and ground truth manual segmentation was provided by an expert radiologist. The proposed method yielded an average Dice score of 93.1 % and Hausdorff distance of 4.2 mm, whereas the U-net yielded 91.6 % and 11.9 mm for Dice score and Hausdorff distance metrics, respectively. The quantitative evaluations demonstrated that the proposed method performed significantly better than U-net in terms of Hausdorff distance in addition to providing open contour-based segmentation for the LA.
- IEEE MemberUS $11.00
- Society MemberUS $0.00
- IEEE Student MemberUS $11.00
- Non-IEEE MemberUS $15.00
CNN in CT Image Segmentation: Beyond Loss Function for Exploiting Ground Truth Images
Exploiting more information from ground truth (GT) images now is a new research direction for further improving CNN's performance in CT image segmentation. Previous methods focus on devising the loss function for fulfilling such a purpose. However, it is rather difficult to devise a general and optimization-friendly loss function. We here present a novel and practical method that exploits GT images beyond the loss function. Our insight is that feature maps of two CNNs trained respectively on GT and CT images should be similar on some metric space, because they both are used to describe the same objects for the same purpose. We hence exploit GT images by enforcing such two CNNs' feature maps to be consistent. We assess the proposed method on two data sets, and compare its performance to several competitive methods. Extensive experimental results show that the proposed method is effective, outperforming all the compared methods.
- IEEE MemberUS $11.00
- Society MemberUS $0.00
- IEEE Student MemberUS $11.00
- Non-IEEE MemberUS $15.00
Topology Highlights Neural Deficits of Post-Stroke Aphasia Patients
Statistical inference of topological features decoded by persistent homology, a topological data analysis (TDA) algorithm, has been found to reveal patterns in electroencephalographic (EEG) signals that are not captured by standard temporal and spectral analysis. However, a potential challenge for applying topological inference to large-scale EEG data is the ambiguity of performing statistical inference and computational bottleneck. To address this problem, we advance a unified permutation-based inference framework for testing statistical difference in the topological feature persistence landscape (PL) of multi-trial EEG signals. In this study, we apply the framework to compare the PLs in EEG signals recorded in participants with aphasia vs. a matched control group during altered auditory feedback tasks.
- IEEE MemberUS $11.00
- Society MemberUS $0.00
- IEEE Student MemberUS $11.00
- Non-IEEE MemberUS $15.00
Generating Controllable Ultrasound Images of the Fetal Head
Synthesis of anatomically realistic ultrasound images could be potentially valuable in sonographer training and to provide training images for algorithms, but is a challenging technical problem. Generating examples where different image attributes can be controlled may also be useful for tasks such as semi-supervised classification and regression to augment costly human annotation. In this paper, we propose using an information maximizing generative adversarial network with a least-squares loss function to generate new examples of fetal brain ultrasound images from clinically acquired healthy subject twenty-week anatomy scans. The unsupervised network succeeds in disentangling natural clinical variations in anatomical visibility and image acquisition parameters, which allows for user-control in image generation. To evaluate our method, we also introduce an additional synthetic fetal ultrasound specific image quality metric called the Frechet SonoNet Distance (FSD) to quantitatively evaluate synthesis quality. To the best of our knowledge, this is the first work that generates ultrasound images with a generator network trained on clinical acquisitions where governing parameters can be controlled in a visually interpretable manner.
- IEEE MemberUS $11.00
- Society MemberUS $0.00
- IEEE Student MemberUS $11.00
- Non-IEEE MemberUS $15.00
Complementary Network with Adaptive Receptive Fields for Melanoma Segmentation
Automatic melanoma segmentation in dermoscopic images is essential in computer-aided diagnosis of skin cancer. Existing methods may suffer from the hole and shrink problems with limited segmentation performance. To tackle these issues, we propose a novel complementary network with adaptive receptive filed learning. Instead of regarding the segmentation task independently, we introduce a foreground network to detect melanoma lesions and a background network to mask non-melanoma regions. Moreover, we propose adaptive atrous convolution (AAC) and knowledge aggregation module (KAM) to fill holes and alleviate the shrink problems. AAC allows us to explicitly control the receptive field at multiple scales. KAM convolves shallow feature maps by dilated convolutions with adaptive receptive fields, which are adjusted according to deep feature maps. In addition, A novel mutual loss is proposed to utilize the dependency between the foreground and background networks, thereby enabling the reciprocally influence within these two networks. Consequently, this mutual training strategy enables the semi-supervised learning and improve the boundary-sensitivity. Training with Skin Imaging Collaboration (ISIC) 2018 skin lesion segmentation dataset, our method achieves a dice coefficient of 86.4% and shows better performance compared with state-of-the-art melanoma segmentation methods.
- IEEE MemberUS $11.00
- Society MemberUS $0.00
- IEEE Student MemberUS $11.00
- Non-IEEE MemberUS $15.00
Probabilistic Inference for Camera Calibration in Light Microscopy under Circular Motion
Robust and accurate camera calibration is essential for 3D reconstruction in light microscopy under circular motion. Conventional methods require either accurate key point matching or precise segmentation of the axial-view images. Both remain challenging because specimens often exhibit transparency/translucency in a light microscope. To address those issues, we propose a probabilistic inference based method for the camera calibration that does not require sophisticated image pre-processing. Based on 3D projective geometry, our method assigns a probability on each of a range of voxels that cover the whole object. The probability indicates the likelihood of a voxel belonging to the object to be reconstructed. Our method maximizes a joint probability that distinguishes the object from the background. Experimental results show that the proposed method can accurately recover camera configurations in both light microscopy and natural scene imaging. Furthermore, the method can be used to produce high-fidelity 3D reconstructions and accurate 3D measurements.
- IEEE MemberUS $11.00
- Society MemberUS $0.00
- IEEE Student MemberUS $11.00
- Non-IEEE MemberUS $15.00
Computer Aided Diagnosis of Clinically Significant Prostate Cancer in Low-Risk Patients on Multi-Parametric Mr Images Using Deep Learning
The purpose of this study was to develop a quantitative method for detection and segmentation of clinically significant (ISUP grade = 2) prostate cancer (PCa) in low-risk patient. A consecutive cohort of 356 patients (active surveillance) was selected and divided in two groups: 1) MRI and targeted-biopsy positive PCa, 2) MRI and standard-biopsy negative PCa. A 3D convolutional neural network was trained in three-fold cross validation with MRI and targeted-biopsy positive patient?s data using two mp-MRI sequences (T2-weighted, DWI-b800) and ADC map as input. After training, the model was tested on separate positive and negative patients to evaluate the performance. The model achieved an average area under the curve (AUC) of the receiver operating characteristics is 0.78 (sensitivity = 85%, specificity = 72%). The diagnostic performance of the proposed method in segmenting significant PCa and to conform non-significant PCa in low-risk patients is characterized by a good AUC and negative-predictive-value.
- IEEE MemberUS $11.00
- Society MemberUS $0.00
- IEEE Student MemberUS $11.00
- Non-IEEE MemberUS $15.00
Using Transfer Learning and Class Activation Maps Supporting Detection and Localization of Femoral Fractures on Anteroposterior Radiographs
Acute Proximal Femoral Fractures are a growing health concern among the aging population. These fractures are often associated with significant morbidity and mortality as well as reduced quality of life. Furthermore, with the increasing life expectancy owing to advances in healthcare, the number of proximal femoral fractures may increase by a factor of 2 to 3, since the majority of fractures occur in patients over the age of 65. In this paper, we show that by using transfer learning and leveraging pre-trained models, we can achieve very high accuracy in detecting fractures and that they can be localized utilizing class activation maps.
- IEEE MemberUS $11.00
- Society MemberUS $0.00
- IEEE Student MemberUS $11.00
- Non-IEEE MemberUS $15.00
Compressed Sensing for Data Reduction in Synthetic Aperture Ultrasound Imaging: A Feasibility Study
Compressed Sensing (CS) has been applied by a few researchers to improve the frame rate of synthetic aperture (SA) ultrasound imaging. However, there appear to be no reports on reducing the number of receive elements by exploiting CS approach. In our previous work, we have proposed a strategic undersampling scheme based on Gaussian distribution for focused ultrasound imaging. In this work, we propose and evaluate three sampling schemes for SA to acquire RF data from a reduced number of receive elements. The effect of sampling schemes on CS recovery was studied using simulation and experimental data. In spite of using only 50% of the receive elements, it was found that the ultrasound images using the Gaussian sampling scheme had comparable resolution and contrast with respect to the reference image obtained using all the receive elements. Thus, the findings suggest a possibility to reduce the receive channel count of SA ultrasound system without practically sacrificing the image quality.
- IEEE MemberUS $11.00
- Society MemberUS $0.00
- IEEE Student MemberUS $11.00
- Non-IEEE MemberUS $15.00
Residual Simplified Reference Tissue Model with Covariance Estimation
The simplified reference tissue model (SRTM) can robustly estimate binding potential (BP) without a measured arterial blood input function. Although a voxel-wise estimation of BP, so-called parametric image, is more useful than the region of interest (ROI) based estimation of BP, it is challenging to calculate the accurate parametric image due to lower signal-to-noise ratio (SNR) of dynamic PET images. To achieve reliable parametric imaging, temporal images are commonly smoothed prior to the kinetic parameter estimation, which degrades the resolution significantly. To address the problem, we propose a residual simplified reference tissue model (ResSRTM) using an approximate covariance matrix to robustly compute the parametric image with a high resolution. We define the residual dynamic data as full data except for each frame data, which has higher SNR and can achieve the accurate estimation of parametric image. Since dynamic images have correlations across temporal frames, we propose an approximate covariance matrix using neighbor voxels by assuming the noise statistics of neighbors are similar. In phantom simulation and real experiments, we demonstrate that the proposed method outperforms the conventional SRTM method.
- IEEE MemberUS $11.00
- Society MemberUS $0.00
- IEEE Student MemberUS $11.00
- Non-IEEE MemberUS $15.00
Spatially Informed Cnn for Automated Cone Detection in Adaptive Optics Retinal Images
Adaptive optics (AO) scanning laser ophthalmoscopy offers cellular level in-vivo imaging of the human cone mosaic. Existing analysis of cone photoreceptor density in AO images require accurate identification of cone cells, which is a time and labor-intensive task. Recently, several methods have been introduced for automated cone detection in AO retinal images using convolutional neural networks (CNN). However, these approaches have been limited in their ability to correctly identify cones when applied to AO images originating from different locations in the retina, due to changes to the reflectance and arrangement of the cone mosaics with eccentricity. To address these limitations, we present an adapted CNN architecture that incorporates spatial information directly into the network. Our approach, inspired by conditional generative adversarial networks, embeds the retina location from which each AO image was acquired as part of the training. Using manual cone identification as ground truth, our evaluation shows general improvement over existing approaches when detecting cones in the middle and periphery regions of the retina, but decreased performance near the fovea.
- IEEE MemberUS $11.00
- Society MemberUS $0.00
- IEEE Student MemberUS $11.00
- Non-IEEE MemberUS $15.00
Deep Mouse: An End-To-End Auto-Context Refinement Framework for Brain Ventricle & Body Segmentation in Embryonic Mice Ultrasound Volumes
The segmentation of the brain ventricle (BV) and body in embryonic mice high-frequency ultrasound (HFU) volumes can provide useful information for biological researchers. However, manual segmentation of the BV and body requires substantial time and expertise. This work proposes a novel deep learning based end-to-end auto-context re?nement framework, consisting of two stages. The ?rst stage produces a low resolution segmentation of the BV and body simultaneously. The resulting probability map for each object (BV or body) is then used to crop a region of interest (ROI) around the target object in both the original image and the probability map to provide context to the re?nement segmentation network. Joint training of the two stages provides signi?cant improvement in Dice Similarity Coef?cient (DSC) over using only the ?rst stage (0.818 to 0.906 for the BV, and 0.919 to 0.934 for the body). The proposed method signi?cantly reduces the inference time (102.36 to 0.09 s/volume ?1000x faster) while slightly improves the segmentation accuracy over the previous methods using slide-window approaches.
- IEEE MemberUS $11.00
- Society MemberUS $0.00
- IEEE Student MemberUS $11.00
- Non-IEEE MemberUS $15.00
Model-Based Deep Learning for Reconstruction of Joint K-Q Under-Sampled High Resolution Diffusion MRI
We propose a model-based deep learning architecture for the reconstruction of highly accelerated diffusion magnetic resonance imaging (MRI) that enables high-resolution imaging. The proposed reconstruction jointly recovers all the diffusion-weighted images in a single step from a joint k-q under-sampled acquisition in a parallel MRI setting. We propose the novel use of a pre-trained denoiser as a regularizer in a model-based reconstruction for the recovery of highly under-sampled data. Specifically, we designed the denoiser based on a general diffusion MRI tissue microstructure model for multi-compartmental modeling. By using a wide range of biologically plausible parameter values for the multi-compartmental microstructure model, we simulated diffusion signal that spans the entire microstructure parameter space. A neural network was trained in an unsupervised manner using a convolutional autoencoder to learn the diffusion MRI signal subspace. We employed the autoencoder in a model-based reconstruction that unrolls the iterations similar to the recently proposed MoDL framework. Specifically, we show that the autoencoder provides a strong denoising prior to recover the q-space signal. We show reconstruction results on a simulated brain dataset that shows high acceleration capabilities of the proposed method.
- IEEE MemberUS $11.00
- Society MemberUS $0.00
- IEEE Student MemberUS $11.00
- Non-IEEE MemberUS $15.00
Deep Learning for High Speed Optical Coherence Elastography
Mechanical properties of tissue provide valuable information for identifying lesions. One approach to obtain quantitative estimates of elastic properties is shear wave elastography with optical coherence elastography (OCE). However, given the shear wave velocity, it is still difficult to estimate elastic properties. Hence, we propose deep learning to directly predict elastic tissue properties from OCE data. We acquire 2D images with a frame rate of 30 kHz and use convolutional neural networks to predict gelatin concentration, which we use as a surrogate for tissue elasticity. We compare our deep learning approach to predictions from conventional regression models, using the shear wave velocity as a feature. Mean absolut prediction errors for the conventional approaches range from 1.32+-0.98 p.p. to 1.57+-1.30 p.p. whereas we report an error of 0.90+-0.84 p.p. for the convolutional neural network with 3D spatio-temporal input. Our results indicate that deep learning on spatio-temporal data outperforms elastography based on explicit shear wave velocity estimation.
- IEEE MemberUS $11.00
- Society MemberUS $0.00
- IEEE Student MemberUS $11.00
- Non-IEEE MemberUS $15.00
Weakly Supervised Multi-Task Learning for Cell Detection and Segmentation
Cell detection and segmentation is fundamental for all downstream analysis of digital pathology images. However, obtaining the pixel-level ground truth for single cell segmentation is extremely labor intensive. To overcome this challenge, we developed an end-to-end deep learning algorithm to perform both single cell detection and segmentation using only point labels. This is achieved through the combination of different task orientated point label encoding methods and a multi-task scheduler for training. We apply and validate our algorithm on PMS2 stained colon rectal cancer and tonsil tissue images. Compared to the state-of-the-art, our algorithm shows significant improvement in cell detection and segmentation without increasing the annotation efforts.
- IEEE MemberUS $11.00
- Society MemberUS $0.00
- IEEE Student MemberUS $11.00
- Non-IEEE MemberUS $15.00
Fully Unsupervised Probabilistic Noise2void
Image denoising is the first step in many biomedical image analysis pipelines and Deep Learning (DL) based methods are currently best performing. A new category of DL methods such as Noise2Void or Noise2Self can be used fully unsupervised, requiring nothing but the noisy data. However, this comes at the price of reduced reconstruction quality. The recently proposed Probabilistic Noise2Void (PN2V) improves results, but requires an additional noise model for which calibration data needs to be acquired. Here, we present improvements to PN2V that (i) replace histogram based noise models by parametric noise models, and (ii) show how suitable noise models can be created even in the absence of calibration data. This is a major step since it actually renders PN2V fully unsupervised. We demonstrate that all proposed improvements are not only academic but indeed relevant.
- IEEE MemberUS $11.00
- Society MemberUS $0.00
- IEEE Student MemberUS $11.00
- Non-IEEE MemberUS $15.00
Temporally Adaptive-Dynamic Sparse Network for Modeling Disease Progression
Alzheimer's disease (AD) is a neurodegenerative disorder with progressive impairment of memory and cognitive functions. Sparse coding (SC) has been demonstrated to be an efficient and effective method for AD diagnosis and prognosis. However, previous SC methods usually focus on the baseline data while ignoring the consistent longitudinal features with strong sparsity pattern along the disease progression. Additionally, SC methods extract sparse features from image patches separately rather than learn with the dictionary atoms across the entire subject. To address these two concerns and comprehensively capture temporal-subject sparse features towards earlier and better discriminability of AD, we propose a novel supervised SC network termed Temporally Adaptive-Dynamic Sparse Network (TADsNet) to uncover the sequential correlation and native subject-level codes from the longitudinal brain images. Our work adaptively updates the sparse codes to impose the temporal regularized correlation and dynamically mine the dictionary atoms to make use of entire subject-level features. Experimental results on ADNI-I cohort validate the superiority of our approach.
- IEEE MemberUS $11.00
- Society MemberUS $0.00
- IEEE Student MemberUS $11.00
- Non-IEEE MemberUS $15.00
Interpreting Age Effects of Human Fetal Brain From Spontaneous FMRI Using Deep 3D Convolutional Neural Networks
Understanding human fetal neurodevelopment is of great clinical importance as abnormal development is linked to adverse neuropsychiatric outcomes after birth. With the advances in functional Magnetic Resonance Imaging (fMRI), recent stud- ies focus on brain functional connectivity and have provided new insight into development of the human brain before birth. Deep Convolutional Neural Networks (CNN) have achieved remarkable success on learning directly from image data, yet have not been applied on fetal fMRI for understanding fetal neurodevelopment. Here, we bridge this gap by applying a novel application of 3D CNN to fetal blood oxygen-level dependence (BOLD) resting-state fMRI data. We build supervised CNN to isolate variation in fMRI signals that relate to younger v.s. older fetal age groups. Sensitivity analysis is then performed to identify brain regions in which changes in BOLD signal are strongly associated with fetal brain age. Based on the analysis, we discovered that regions that most strongly differentiate groups are largely bilateral, share similar distribution in older and younger age groups, and are areas of heightened metabolic activity in early human development.
- IEEE MemberUS $11.00
- Society MemberUS $0.00
- IEEE Student MemberUS $11.00
- Non-IEEE MemberUS $15.00
Automatic Brain Organ Segmentation with 3d Fully Convolutional Neural Network for Radiation Therapy Treatment Planning
3D organ contouring is an essential step in radiation therapy treatment planning for organ dose estimation as well as for optimizing plans to reduce organs-at-risk doses. Manual contouring is time-consuming and its inter-clinician variability adversely affects the outcomes study. Such organs also vary dramatically on sizes --- up to two orders of magnitude difference in volumes. In this paper, we present BrainSegNet, a novel 3D fully convolutional neural network (FCNN) based approach for the automatic segmentation of brain organs. BrainSetNet takes a multiple resolution paths approach and uses a weighted loss function to solve the major challenge of large variability in organ sizes. We evaluated our approach with a dataset of 46 Brain CT image volumes with corresponding expert organ contours as reference. Compared with those of LiviaNet and V-Net, BrainSegNet has a superior performance in segmenting tiny or thin organs, such as chiasm, optic nerves, and cochlea, and outperforms these methods in segmenting large organs as well. BrainSegNet can reduce the manual contouring time of a volume from an hour to less than two minutes, and holds high potential to improve the efficiency of radiation therapy workflow.
- IEEE MemberUS $11.00
- Society MemberUS $0.00
- IEEE Student MemberUS $11.00
- Non-IEEE MemberUS $15.00
Breast Lesion Segmentation in Ultrasound Images with Limited Annotated Data
Ultrasound (US) is one of the most commonly used imaging modalities in both diagnosis and surgical interventions due to its low-cost, safety, and non-invasive characteristic. US image segmentation is currently a unique challenge because of the presence of speckle noise. As manual segmentation requires considerable efforts and time, the development of automatic segmentation algorithms has attracted researchers? attention. Although recent methodologies based on convolutional neural networks have shown promising performances, their success relies on the availability of a large number of training data, which is prohibitively difficult for many applications. There- fore, in this study we propose the use of simulated US images and natural images as auxiliary datasets in order to pre-train our segmentation network, and then to fine-tune with limited in vivo data. We show that with as little as 19 in vivo images, fine-tuning the pre-trained network improves the dice score by 21% compared to training from scratch. We also demonstrate that if the same number of natural and simulation US images is available, pre-training on simulation data is preferable.
- IEEE MemberUS $11.00
- Society MemberUS $0.00
- IEEE Student MemberUS $11.00
- Non-IEEE MemberUS $15.00
7t Guided 3t Brain Tissue Segmentation Using Cascaded Nested Network
Accurate segmentation of the brain into major tissue types, e.g., the gray matter, white matter, and cerebrospinal fluid, in magnetic resonance (MR) imaging is critical for quantification of the brain anatomy and function. The availability of 7T MR scanners can provide more accurate and reliable voxel-wise tissue labels, which can be leveraged to supervise the training of the tissue segmentation in the conventional 3T brain images. Specifically, a deep learning based method can be used to build the highly non-linear mapping from the 3T intensity image to the more reliable label maps obtained from the 7T images of the same subject. However, the misalignment between 3T and 7T MR images due to image distortions poses a major obstacle to achieving better segmentation accuracy. To address this issue, we measure the quality of the 3T-7T alignment by using a correlation coefficient map. Then we propose a cascaded nested network (CaNes-Net) for 3T MR image segmentation and a multi-stage solution for training this model with the ground-truth tissue labels from 7T images. This paper has two main contributions. First, by incorporating the correlation loss, the above mentioned obstacle can be well addressed. Second, the geodesic distance maps are constructed based on the intermediate segmentation results to guide the training of the CaNes-Net as an iterative coarse-to-fine process. We evaluated the proposed CaNes-Net with the state-of-the-art methods on 18 in-house acquired subjects. We also qualitatively assessed the performance of the proposed model and U-Net on the ADNI dataset. Our results indicate that the proposed CaNes-Net is able to dramatically reduce mis-segmentation caused by the misalignment and achieves substantially improved accuracy over all the other methods.
- IEEE MemberUS $11.00
- Society MemberUS $0.00
- IEEE Student MemberUS $11.00
- Non-IEEE MemberUS $15.00
Sex differences in the brain: Divergent results from traditional machine learning and convolutional networks
Neuroimaging research has begun adopting deep learning to model structural differences in the brain. This is a break from previous approaches that rely on derived features from brain MRI, such as regional thicknesses or volumes. To date, most studies employ either deep learning based models or traditional machine learning volume based models. Because of this split, it is unclear which approach is yielding better predictive performance or if the two approaches will lead to different neuroanatomical conclusions, potentially even when applied to the same datasets. In the present study, we carry out the largest single study of sex differences in the brain using 21,390 UK Biobank T1-weighted brain MRIs analyzed through both traditional and 3D convolutional neural network models. Through comparing performances, we find that 3D-CNNs outperform traditional machine learning models using volumetric features. Through comparing regions highlighted by both approaches, we find poor overlap in conclusions derived from traditional machine learning and 3D-CNN based models. In summary, we find that 3D-CNNs show exceptional predictive performance, but may highlight neuroanatomical regions different from what would be found by volume-based approaches.
- IEEE MemberUS $11.00
- Society MemberUS $0.00
- IEEE Student MemberUS $11.00
- Non-IEEE MemberUS $15.00
A Novel End-To-End Hybrid Network for Alzheimers Disease Detection Using 3D CNN and 3D CLSTM
Structural magnetic resonance imaging (sMRI) plays an important role in Alzheimer?s disease (AD) detection as it shows morphological changes caused by brain atrophy. Convolutional neural network (CNN) has been successfully used to achieve good performance in accurate diagnosis of AD. However, most existing methods utilized shallow CNN structures due to the small amount of sMRI data, which limits the ability of CNN to learn high-level features. Thus, in this paper, we propose a novel unified CNN framework for AD identification, where both 3D CNN and 3D convolutional long short-term memory (3D CLSTM) are employed. Specifically, we firstly exploit a 6-layer 3D CNN to learn informative features, then 3D CLSTM is leveraged to further extract the channel-wise higher-level information. Extensive experimental results on ADNI dataset show that our model has achieved an accuracy of 94.19% for AD detection, which outperforms the state-of-the-art methods and indicates the high effectiveness of our proposed method
- IEEE MemberUS $11.00
- Society MemberUS $0.00
- IEEE Student MemberUS $11.00
- Non-IEEE MemberUS $15.00
Deep Learning Method for Intracranial Hemorrhage Detection and Subtype Differentiation
Early and accurate diagnosis of Intracranial Hemorrhage (ICH) has a great clinical significance for timely treatment. In this study, we proposed a deep learning method for automatic ICH diagnosis. We exploited three windowing levels to enhance different tissue contrasts to be used for feature extraction. Our convolutional neural network (CNN) model employed the EfficientNet-B2 architecture and was re-trained using a published annotated computer tomography (CT) image dataset of ICH. The performance of our model has the overall accuracy of 0.973 and precision of 0.965. The processing time is less than 0.5 second per image slice.
- IEEE MemberUS $11.00
- Society MemberUS $0.00
- IEEE Student MemberUS $11.00
- Non-IEEE MemberUS $15.00
A Deep Learning Framework to Expedite Infrared Spectroscopy for Digital Histopathology
Histopathology, based on examining the morphology of epithelial cells, is the gold standard in clinical diagnosis and research for detecting carcinomas. This is a time-consuming, error-prone, and non-quantitative process. An alternate approach, Fourier transform infrared (FTIR) spectroscopic imaging offers label-free visualization of the tissues by providing spatially-localized chemical information coupled to computational algorithms to reveal contrast between different cell types and diseases, thereby skipping the manual and laborious process of traditional histopathology. While FTIR imaging provides reliable analytical information over a wide spectral profile, data acquisition time is a major challenge in the translation to clinical research. In the acquisition of spectroscopic imaging data, there is an ever-present trade-off between the amount of data recorded and the acquisition time. Since not all the spectral elements are needed for classification, discrete frequency infrared (DFIR) imaging has been introduced to expedite the data recording by measuring required spectral elements. We report a deep learning-based framework to further accelerate the whole process of data acquisition and analysis through also subsampling in the spatial domain. First, we introduce a convolutional neural network (CNN) to leverage both spatial and spectral information for segmenting infrared data, which we term the IRSEG network. We show that this framework increases the accuracy while utilizing approximately half the number of unique bands commonly required for previous pixel-wise classification algorithms used in the DFIR community. Finally, we present a data reconstruction approach using generative adversarial network (GAN) to reconstruct the whole spatial and spectral domain while only using a small fraction of the total possible data, with minimal information loss. We name this IR GAN-based data reconstruction IRGAN. Together, this study paves the way the translation of IR imaging to clinic for label-free histological analysis by boosting the process approximately 20 times faster from hours to minutes.
- IEEE MemberUS $11.00
- Society MemberUS $0.00
- IEEE Student MemberUS $11.00
- Non-IEEE MemberUS $15.00
Detection of Foreign Objects in Chest Radiographs using Deep Learning
We propose a deep learning framework for the automated detection of foreign objects in chest radiographs. Foreign objects can affect the diagnostic quality of an image and could affect the performance of CAD systems. Their automated detection could alert the technologists to take corrective actions. In addition, the detection of foreign objects such as pacemakers or placed devices could also help automate clinical workflow. We used a subset of the MIMIC CXR dataset and annotated 6061 images for six foreign object categories namely tubes and wires, pacemakers, implants, small external objects, jewelry and push-buttons. A transfer learning based approach was developed for both binary and multi-label classification. All networks were pre-trained using the computer vision database ImageNet and the NIH database ChestX-ray14. The evaluation was performed using 5-fold cross-validation (CV) and an additional test set with 1357 images. We achieved the best average area under the ROC curve (AUC) of 0.972 for binary classification and 0.969 for multilabel classification using 5-fold CV. On the test dataset, the respective best AUCs of 0.984 and 0.969 were obtained using a dense convolutional network.
- IEEE MemberUS $11.00
- Society MemberUS $0.00
- IEEE Student MemberUS $11.00
- Non-IEEE MemberUS $15.00
Lung Ct Screening With 3D Convolutional Neural Network Architecture
Lung cancer is the most prevalent cancer in the world and early detection and diagnosis enable more treatment options and a far greater chance of survival. Computer-aided detection systems can be used to assist specialists providing a second opinion of the analysis in the detection of pulmonary nodules. Thus, we propose an algorithm based on 3D Convolutional Neural Network to classify pulmonary nodules as benign or malignant from Computed Tomography images. The proposed architecture has two blocks of convolutional layers followed by a pooling layer, two fully connected layers and a softmax layer that represents the network output. The results show an accuracy of 91.60% and an error of 0.2761 in the test set. These are promising results for the application of 3D CNN in the detection of malignant nodules.
- IEEE MemberUS $11.00
- Society MemberUS $0.00
- IEEE Student MemberUS $11.00
- Non-IEEE MemberUS $15.00
Motile cilia and left-right symmetry breaking: from images to biological insights
In vertebrate embryos, cilia-driven fluid flows generated within the left-right organizer (LRO) are guiding the establishment of the left-right body asymmetry. To study such complex and dynamic biomechanical process and to investigate the generation and sensing of biological flows, it is required to quantify biophysical features of motile cilia in 3D and in vivo. In the zebrafish embryo, the LRO is called the Kupffer?s vesicle and is a spheroid shape cavity, which is covered with motile cilia oriented in all directions of space. This dynamic and transient structure varies in size and shape during development and from one embryo to the other. In addition, micrometric size cilia are beating too fast and are located too deep inside the embryo to be able to image their 3D motion pattern using fluorescence microscopy. As a consequence, the experimental investigation of motile cilia properties is challenging. In this talk, we will present how we circumvented these limitations by combining live 3D imaging using multiphoton microscopy and image registration, processing and analysis. We quantified cilia biophysical features, such as density, motility, 3D orientation, beating frequency, or length without resolving their motion. We combined the results from different embryos in order to perform statistical analyses and compare experimental conditions. We integrated such experimental features obtained in vivo into a fluid dynamics model and a multiscale physical study of flow generation and detection. Finally, this strategy enabled us to demonstrate how cilia orientation pattern generate the asymmetric flow within the LRO. In addition, we investigated the physical limits of flow detection to clarify which mechanisms could be reliably used for body axis symmetry breaking. We also identified a novel type of asymmetry in the left-right organizer. Together, this work based on quantitative image analysis of motile cilia sheds light on the complexity of left-right symmetry breaking and chirality genesis in developing tissues.
- IEEE MemberUS $11.00
- Society MemberUS $0.00
- IEEE Student MemberUS $11.00
- Non-IEEE MemberUS $15.00
Unsupervised Task Design to Meta-Train Medical Image Classifiers
Meta-training has been empirically demonstrated to be the most effective pre-training method for few-shot learning of medical image classifiers (i.e., classifiers modeled with small training sets). However, the effectiveness of meta-training relies on the availability of a reasonable number of hand-designed classification tasks, which are costly to obtain, and consequently rarely available. In this paper, we propose a new method to unsupervisedly design a large number of classification tasks to meta-train medical image classifiers. We evaluate our method on a breast dynamically contrast enhanced magnetic resonance imaging (DCE-MRI) data set that has been used to benchmark few-shot training methods of medical image classifiers. Our results show that the proposed unsupervised task design to meta-train medical image classifiers builds a pre-trained model that, after fine-tuning, produces better classification results than other unsupervised and supervised pre-training methods, and competitive results with respect to meta-training that relies on hand-designed classification tasks.
- IEEE MemberUS $11.00
- Society MemberUS $0.00
- IEEE Student MemberUS $11.00
- Non-IEEE MemberUS $15.00
Volumetric Landmark Detection with a Multi-Scale Shift Equivariant Neural Network
Deep neural networks yield promising results in a wide range of computer vision applications, including landmark detection. A major challenge for accurate anatomical landmark detection in volumetric images such as clinical CT scans is that large-scale data often constrain the capacity of the employed neural network architecture due to GPU memory limitations, which in turn can limit the precision of the output. We propose a multi-scale, end-to-end deep learning method that achieves fast and memory-efficient landmark detection in 3D images. Our architecture consists of blocks of shift-equivariant networks, each of which performs landmark detection at a different spatial scale. These blocks are connected from coarse to fine-scale, with differentiable resampling layers, so that all levels can be trained together. We also present a noise injection strategy that increases the robustness of the model and allows us to quantify uncertainty at test time. We evaluate our method for carotid artery bifurcations detection on 263 CT volumes and achieve a better than state-of-the-art accuracy with mean Euclidean distance error of 2.81mm.
- IEEE MemberUS $11.00
- Society MemberUS $0.00
- IEEE Student MemberUS $11.00
- Non-IEEE MemberUS $15.00
Robust Algorithm for Denoising of Photon-Limited Dual-Energy Cone Beam CT Projections
Dual-Energy CT offers significant advantages over traditional CT imaging because it offers energy-based awareness of the image content and facilitates material discrimination in the projection domain. The Dual-Energy CT concept has intrinsic redundancy that can be used for improving image quality, by jointly exploiting the high- and low-energy projections. In this paper we focus on noise reduction. This work presents the novel noise-reduction algorithm Dual Energy Shifted Wavelet Denoising (DESWD), which renders high-quality Dual-Energy CBCT projections out of noisy ones. To do so, we first apply a Generalized Anscombe Transform, enabling us to use denoising methods proposed for Gaussian noise statistics. Second, we use a 3D transformation to denoise all the projections at once. Finally we exploit the inter-channel redundancy of the projections to create sparsity in the signal for better denoising with a channel-decorrelation step. Our simulation experiments show that DESWD performs better than a state-of-the-art denoising method (BM4D) in limited photon-count imaging, while BM4D achieves excellent results for less noisy conditions.
- IEEE MemberUS $11.00
- Society MemberUS $0.00
- IEEE Student MemberUS $11.00
- Non-IEEE MemberUS $15.00
Multi-modality Generative Adversarial Networks with Humor Consistency Loss for Brain MR Image Synthesis
Magnetic Resonance (MR) images of different modalities can provide complementary information for clinical diagnosis, but whole modalities are often costly to access. Most existing methods only focus on synthesizing missing images between two modalities, which limits their robustness and efficiency when multiple modalities are missing. To address this problem, we propose a multi-modality generative adversarial network (MGAN) to synthesize three high-quality MR modalities (FLAIR, T1 and T1ce) from one MR modality T2 simultaneously. The experimental results show that the quality of the synthesized images by our proposed methods is better than the one synthesized by the baseline model, pix2pix. Besides, for MR brain image synthesis, it is important to preserve the critical tumor information in the generated modalities, so we further introduce a multi-modality tumor consistency loss to MGAN, called TC-MGAN. We use the synthesized modalities by TC-MGAN to boost the tumor segmentation accuracy, and the results demonstrate its effectiveness.
- IEEE MemberUS $11.00
- Society MemberUS $0.00
- IEEE Student MemberUS $11.00
- Non-IEEE MemberUS $15.00
Volumetric Registration-Based Cleft Volume Estimation of Alveolar Cleft Grafting Procedures
This paper presents a method for automatic estimation of the bony alveolar cleft volume of cleft lips and palates (CLP) patients from cone-beam computed tomography (CBCT) images via a fully convolutional neural network. The core of this method is the partial nonrigid registration of the CLP CBCT image with the incomplete maxilla and the template with the complete maxilla. We build our model on the 3D U-Net and parameterize the nonlinear mapping from the one-channel intensity CBCT image to six-channel inverse deformation vector fields (DVF). We enforce the partial maxillary registration using an adaptive irregular mask regarding the cleft in the registration process. When given inverse DVFs, the deformed template combined with volumetric Boolean operators are used to compute the cleft volume. To avoid the rough and inaccurate reconstructed cleft surface, we introduce an additional cleft shape constraint to fine-tune the parameters of the registration neural networks. The proposed method is applied to clinically-obtained CBCT images of CLP patients. The qualitative and quantitative experiments demonstrate the effectiveness and efficiency of our method in the volume completion and the bony cleft volume estimation compared with the state-of-the-art.
- IEEE MemberUS $11.00
- Society MemberUS $0.00
- IEEE Student MemberUS $11.00
- Non-IEEE MemberUS $15.00
Unsupervised Adversarial Correction of Rigid MR Motion Artifacts
Motion is one of the main sources for artifacts in magnetic resonance (MR) images. It can have significant consequences on the diagnostic quality of the resultant scans. Previously, supervised adversarial approaches have been suggested for the correction of MR motion artifacts. However, these approaches suffer from the limitation of required paired co-registered datasets for training which are often hard or impossible to acquire. Building upon our previous work, we introduce a new adversarial framework with a new generator architecture and loss function for the unsupervised correction of severe rigid motion artifacts in the brain region. Quantitative and qualitative comparisons with other supervised and unsupervised translation approaches showcase the enhanced performance of the introduced framework.
- IEEE MemberUS $11.00
- Society MemberUS $0.00
- IEEE Student MemberUS $11.00
- Non-IEEE MemberUS $15.00
Annotation-Free Gliomas Segmentation Based on a Few Labeled General Brain Tumor Images
Pixel-level labeling for medical image segmentation is time-consuming and sometimes infeasible. Therefore, using a small amount of labeled data in one domain to help train a reasonable segmentation model for unlabeled data in another domain becomes an important need in medical image segmentation. In this work, we propose a new segmentation framework based on unsupervised domain adaptation and semi-supervised learning, which uses a small amount of labeled general brain tumor images and learns an effective model to segment independent brain gliomas images. Our method contains two major parts. First, we use unsupervised domain adaptation to generate synthetic general brain tumor images from the brain gliomas images. Then, we apply semi-supervised learning method to train a segmentation model with a small number of labeled general brain tumor images and the unlabeled synthetic images. The experimental results show that our proposed method can use approximate 10% of labeled data to achieve a comparable accuracy of the model trained with all labeled data.
- IEEE MemberUS $11.00
- Society MemberUS $0.00
- IEEE Student MemberUS $11.00
- Non-IEEE MemberUS $15.00
3D Optical Flow Estimation Combining 3D Census Signature and Total Variation Regularization
We present a 3D variational optical flow method for fluorescence image sequences which preserves discontinuities in the computed flow field. We propose to minimize an energy function composed of a linearized 3D Census signature-based data term and a total variational (TV) regularizer. To demonstrate the efficiency of our method, we have applied real sequences depicting collagen network, where the motion field is expected to be discontinuous. We also favorably compare our results with two other motion estimation methods.
- IEEE MemberUS $11.00
- Society MemberUS $0.00
- IEEE Student MemberUS $11.00
- Non-IEEE MemberUS $15.00
Low-Dose Cardiac-Gated Spect Via a Spatiotemporal Convolutional Neural Network
In previous studies convolutional neural networks (CNN) have been demonstrated to be effective for suppressing the elevated imaging noise in low-dose single-photon emission computed tomography (SPECT). In this study, we investigate a spatiotemporal CNN model (ST-CNN) to exploit the signal redundancy in both spatial and temporal domains among the gate frames in a cardiac-gated sequence. In the experiments, we demonstrated the proposed ST-CNN model on a set of 119 clinical acquisitions with imaging dose reduced by four times. The quantitative results show that ST-CNN can lead to further improvement in the reconstructed myocardium in terms of the overall error level and the spatial resolution of the left ventricular (LV) wall. Compared to a spatial-only CNN, STCNN decreased the mean-squared-error of the reconstructed myocardium by 21.1% and the full-width at half-maximum of the LV wall by 5.3%.
- IEEE MemberUS $11.00
- Society MemberUS $0.00
- IEEE Student MemberUS $11.00
- Non-IEEE MemberUS $15.00
Spectral Data Augmentation Techniques to Quantify Lung Pathology from CT-Images
Data augmentation is of paramount importance in biomedical image processing tasks, characterized by inadequate amounts of labelled data, to best use all of the data that is present. In-use techniques range from intensity transformations and elastic deformations, to linearly combining existing data points to make new ones. In this work, we propose the use of spectral techniques for data augmentation, using the discrete cosine and wavelet transforms. We empirically evaluate our approaches on a CT texture analysis task to detect abnormal lung-tissue in patients with cystic fibrosis. Empirical experiments show that the proposed spectral methods perform favourably as compared to the existing methods. When used in combination with existing methods, our proposed approach can increase the relative minor class segmentation performance by 44.1% over a simple replication baseline.
- IEEE MemberUS $11.00
- Society MemberUS $0.00
- IEEE Student MemberUS $11.00
- Non-IEEE MemberUS $15.00
Unsupervised Learning of Contextual Information in Multiplex Immunofluorescence Tissue Cytometry
New machine learning models designed to capture the histopathology of tissues should account not only for the phenotype and morphology of the cells, but also learn complex spatial relationships between them. To achieve this, we represent the tissue as an interconnected graph, where previously segmented cells become nodes of the graph. Then the relationships between cells are learned and embedded into a low-dimensional vector, using a Graph Neural Network. We name this Representation Learning based strategy NARO (NAtural Representation of biological Objects), a fully-unsupervised method that learns how to optimally encode cell phenotypes, morphologies, and cell-to-cell interactions from histological tissues labeled using multiplex immunohistochemistry. To validate NARO, we first use synthetically generated tissues to show that NARO?s generated embeddings can be used to cluster cells in meaningful, distinct anatomical regions without prior knowledge of constituent cell types and interactions. Then we test NARO on real multispectral images of human lung adenocarcinoma tissue samples, to show that the generated embeddings can indeed be used to automatically infer regions with different histopathological characteristics.
- IEEE MemberUS $11.00
- Society MemberUS $0.00
- IEEE Student MemberUS $11.00
- Non-IEEE MemberUS $15.00
A Completion Network for Reconstruction from Compressed Acquisition
We consider here the problem of reconstructing an image from a few linear measurements. This problem has many biomedical applications, such as computerized tomography, magnetic resonance imaging and optical microscopy. While this problem has long been solved by compressed sensing methods, these are now outperformed by deep-learning approaches. However, understanding why a given network architecture works well is still an open question. In this study, we proposed to interpret the reconstruction problem as a Bayesian completion problem where the missing measurements are estimated from those acquired. From this point of view, a network emerges that includes a fully connected layer that provides the best linear completion scheme. This network has a lot fewer parameters to learn than direct networks, and it trains more rapidly than image-domain networks that correct pseudo inverse solutions. Although, this study focuses on computational optics, it might provide some insight for inverse problems that have similar formulations.
- IEEE MemberUS $11.00
- Society MemberUS $0.00
- IEEE Student MemberUS $11.00
- Non-IEEE MemberUS $15.00
Transcriptome-Supervised Classification of Tissue Morphology Using Deep Learning
Deep learning has proven to successfully learn variations in tissue and cell morphology. Training of such models typically relies on expensive manual annotations. Here we conjecture that spatially resolved gene expression, e.i., the transcriptome, can be used as an alternative to manual annotations. In particular, we trained five convolutional neural networks with patches of different size extracted from locations defined by spatially resolved gene expression. The network is trained to classify tissue morphology related to two different genes, general tissue, as well as background, on an image of fluorescence stained nuclei in a mouse brain coronal section. Performance is evaluated on an independent tissue section from a different mouse brain, reaching an average Dice score of 0.51. Results may indicate that novel techniques for spatially resolved transcriptomics together with deep learning may provide a unique and unbiased way to find genotype-phenotype relationships.
- IEEE MemberUS $11.00
- Society MemberUS $0.00
- IEEE Student MemberUS $11.00
- Non-IEEE MemberUS $15.00
Compensatory Brain Connection Discovery in Alzheimers Disease
Identification of the specific brain networks that are vulnerable or resilient in neurodegenerative diseases can help to better understand the disease effects and derive new connectomic imaging biomarkers. In this work, we use brain connectivity to find pairs of structural connections that are negatively correlated with each other across Alzheimer?s disease (AD) and healthy populations. Such anti-correlated brain connections can be informative for identification of compensatory neuronal pathways and the mechanism of brain networks? resilience to AD. We find significantly anti-correlated connections in a public diffusion-MRI database, and then validate the results on other databases.
- IEEE MemberUS $11.00
- Society MemberUS $0.00
- IEEE Student MemberUS $11.00
- Non-IEEE MemberUS $15.00
Spectral Graph Transformer Networks for Brain Surface Parcellation
The analysis of the brain surface modeled as a graph mesh is a challenging task. Conventional deep learning approaches often rely on data lying in the Euclidean space. As an extension to irregular graphs, convolution operations are defined in the Fourier or spectral domain. This spectral domain is obtained by decomposing the graph Laplacian, which captures relevant shape information. However, the spectral decomposition across different brain graphs causes inconsistencies between the eigenvectors of individual spectral domains, causing the graph learning algorithm to fail. Current spectral graph convolution methods handle this variance by separately aligning the eigenvectors to a reference brain in a slow iterative step. This paper presents a novel approach for learning the transformation matrix required for aligning brain meshes using a direct data-driven approach. Our alignment and graph processing method provides a fast analysis of brain surfaces. The novel Spectral Graph Transformer (SGT) network proposed in this paper uses very few randomly sub-sampled nodes in the spectral domain to learn the alignment matrix for multiple brain surfaces. We validate the use of this SGT network along with a graph convolution network to perform cortical parcellation. Our method on 101 manually-labeled brain surfaces shows improved parcellation performance over a no-alignment strategy, gaining a significant speed (1400 fold) over traditional iterative alignment approaches.
- IEEE MemberUS $11.00
- Society MemberUS $0.00
- IEEE Student MemberUS $11.00
- Non-IEEE MemberUS $15.00
Automated Hemorrhage Detection from Coarsely Annotated Fundus Images in Diabetic Retinopathy
In this paper, we proposed and validated a novel and effective pipeline for automatically detecting hemorrhage from coarsely-annotated fundus images in diabetic retinopathy. The proposed framework consisted of three parts: image preprocessing, training data refining, and object detection using a convolutional neural network with label smoothing. Contrast limited adaptive histogram equalization and adaptive gamma correction with weighting distribution were adopted to improve image quality by enhancing image contrast and correcting image illumination. To refine coarsely-annotated training data, we designed a bounding box refining network (BBR-net) to provide more accurate bounding box annotations. Combined with label smoothing, RetinaNet was implemented to alleviate mislabeling issues and automatically detect hemorrhages. The proposed method was trained and evaluated on a publicly available IDRiD dataset and also one of our private datasets. Experimental results showed that our BBR-net could effectively refine manually-delineated coarse hemorrhage annotations, with the average IoU being 0.8715 when compared with well-annotated bounding boxes. The proposed hemorrhage detection pipeline was compared to several alternatives and superior performance was observed.
- IEEE MemberUS $11.00
- Society MemberUS $0.00
- IEEE Student MemberUS $11.00
- Non-IEEE MemberUS $15.00
Unsupervised Cone-Beam Artifact Removal using CycleGAN and Spectral Blending for Adaptive Radiotherapy
Cone-beam computed tomography (CBCT) used in radiotherapy (RT) has the advantage of being taken daily, but is difficult to use for purposes other than patient setup because of the poor image quality compared to fan-beam computed tomography (CT). Even though several methods have been proposed including the deformable image registration method to improve the quality of CBCT, the outcomes have not yet been satisfactory. Recently, deep learning has shown to produce high-quality results for various image-to-image translation tasks, suggesting the possibility of being an effective tool for converting CBCT into CT. In the field of RT, however, it may not always be possible to obtain paired datasets which consist of exactly matching CBCT and CT images. This study aimed to develop a novel, unsupervised deep-learning algorithm, which requires only unpaired CBCT and fan-beam CT images to remove the cone-beam artifact and thereby improve the quality of CBCT. Specifically, two cycle consistency generative adversarial networks (CycleGAN) were trained in the sagittal and coronal directions, and the generated results along those directions were then combined using spectral blending technique. To evaluate our methods, we applied it to American Association of Physicists in Medicine dataset. The experimental results show that our method outperforms the existing CylceGAN-based method both qualitatively and quantitatively.
- IEEE MemberUS $11.00
- Society MemberUS $0.00
- IEEE Student MemberUS $11.00
- Non-IEEE MemberUS $15.00
Macular GCIPL Thickness Map Prediction via Time-Aware Convolutional LSTM
Macular ganglion cell inner plexiform layer (GCIPL) thickness is an important biomarker for clinical managements of glaucoma. Clinical analysis of GCIPL progression uses averaged thickness only, which easily washes out small changes and reveals no spatial patterns. This is the first work to predict the 2D GCIPL thickness map. We propose a novel Time-aware Convolutional Long Short-Term Memory (TC-LSTM) unit to decompose memories into the short-term and long-term memories and exploit time intervals to penalize the short-term memory. TC-LSTM unit is incorporated into an auto-encoder-decoder so that the end-to-end model can handle irregular sampling intervals of longitudinal GCIPL thickness map sequences and capture both spatial and temporal correlations. Experiments show the superiority of the proposed model over the traditional method.
- IEEE MemberUS $11.00
- Society MemberUS $0.00
- IEEE Student MemberUS $11.00
- Non-IEEE MemberUS $15.00
Zero-Shot Medical Image Artifact Reduction
Medical images may contain various types of artifacts with different patterns and mixtures, which depend on many factors such as scan setting, machine condition, patients' characteristics, surrounding environment, etc. However, existing deep learning based artifact reduction methods are restricted by their training set with specific predetermined artifact type and pattern. As such, they have limited clinical adoption. In this paper, we introduce a "Zero-Shot" medical image Artifact Reduction (ZSAR) framework, which leverages the power of deep learning but without using general pre-trained networks or any clean image reference. Specifically, we utilize the low internal visual entropy of an image and train a light-weight image-specific artifact reduction network to reduce artifacts in an image at test-time. We use Computed Tomography (CT) and Magnetic Resonance Imaging (MRI) as vehicles to show that ZSAR can reduce artifacts better than the state-of-the-art both qualitatively and quantitatively, while using shorter test time. To the best of our knowledge, this is the first deep learning framework that reduces artifacts in medical images without using a priori training set.
- IEEE MemberUS $11.00
- Society MemberUS $0.00
- IEEE Student MemberUS $11.00
- Non-IEEE MemberUS $15.00
Functional Multi-Connectivity: A Novel Approach to Assess Multi-Way Entanglement between Networks and Voxels
The interactions among brain entities, commonly computed through pair-wise functional connectivity, are assumed to be manifestations of information processing which drive function. However, this focus on large-scale networks and their pair-wise temporal interactions is likely missing important information contained within fMRI data. We propose leveraging multi-connected features at both the voxel- and network-level to capture ?multi-way entanglement? between networks and voxels, providing improved resolution of interconnected brain functional hierarchy. Entanglement refers to each brain network being heavily enmeshed with the activity of other networks. Under our multi-connectivity assumption, elements of a system simultaneously communicate and interact with each other through multiple pathways. As such we move beyond the typical pair-wise temporal partial or full correlation. We propose a framework to estimate functional multi-connectivity (FMC) by computing the relationship between system-wide connections of intrinsic connectivity networks (ICNs). Results show that FMC obtains information which is different from standard pair-wise analyses.